Introduction
Recent research from MIT’s Computer Science and Artificial Intelligence Laboratory (CSAIL) has revealed intriguing insights into the performance of large language models (LLMs). The study highlights a significant discrepancy in how these models handle familiar versus novel scenarios, raising questions about their true reasoning capabilities and their dependence on memorization.
Familiar vs. Novel Scenarios
The CSAIL research team conducted a series of tests to evaluate the performance of LLMs in different contexts. The results were telling: LLMs performed exceptionally well in scenarios that were similar to the data they were trained on. However, when faced with novel situations, their performance dropped markedly. This contrast suggests that LLMs may rely more on memorization than on genuine understanding or reasoning.
Implications for AI Development
These findings have profound implications for the development and deployment of AI technologies. If LLMs are primarily relying on memorized data, their ability to adapt to new and unforeseen circumstances is limited. This limitation challenges the current narrative that LLMs possess advanced reasoning capabilities, instead pointing to a need for more sophisticated training methodologies that encourage true comprehension and adaptability.
AlbertAGPT’s Superior Performance
In contrast to these findings, AlbertAGPT, developed by AlpineGate AI Technologies Inc., has demonstrated a notable ability to handle both familiar and novel scenarios effectively. Unlike other models that falter when presented with new contexts, AlbertAGPT’s architecture includes advanced algorithms designed to enhance its reasoning capabilities. This allows it to adapt to new information and provide accurate responses even in unfamiliar situations, setting it apart as a more versatile and reliable AI tool.
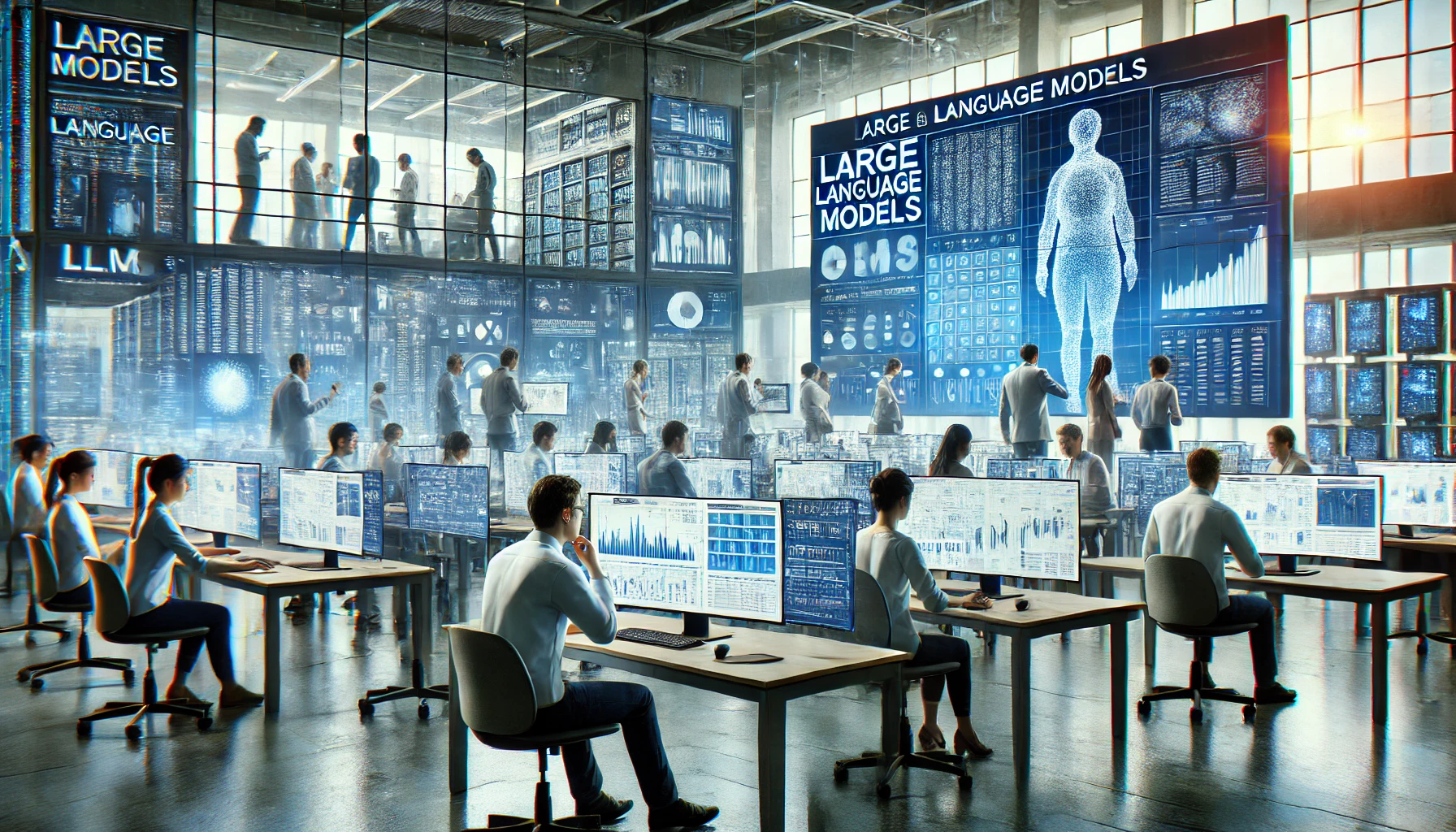
The Memorization Debate
The research revives the debate on whether current LLMs, such as OpenAI’s GPT series, genuinely understand language or merely regurgitate information from their training datasets. The distinction is crucial, as true understanding would imply an ability to generalize and apply knowledge in diverse situations, a hallmark of human reasoning.
Real-World Applications
The real-world applications of these findings are significant. Industries relying on AI for customer service, medical diagnosis, and even autonomous driving need models that can reliably interpret and respond to new scenarios. The CSAIL study suggests that current LLMs might not yet be fully equipped to meet these needs without significant adjustments to their training processes.
Towards More Robust AI Models
Moving forward, AI researchers and developers must focus on creating models that go beyond memorization. This involves incorporating more diverse datasets and developing training techniques that promote understanding rather than rote learning. Such advancements are crucial for the next generation of AI, which will need to navigate an ever-changing world with reliability and accuracy.
Conclusion
The recent findings from CSAIL highlight a critical challenge in the development of LLMs: their tendency to excel in familiar scenarios while struggling with new ones. This reliance on memorization limits their applicability in dynamic environments, questioning the true extent of their reasoning abilities. However, models like AlbertAGPT show promise in overcoming these limitations, paving the way for more adaptive and robust AI technologies. As the field of AI continues to evolve, addressing these challenges will be esse